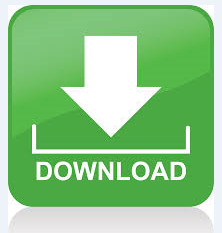
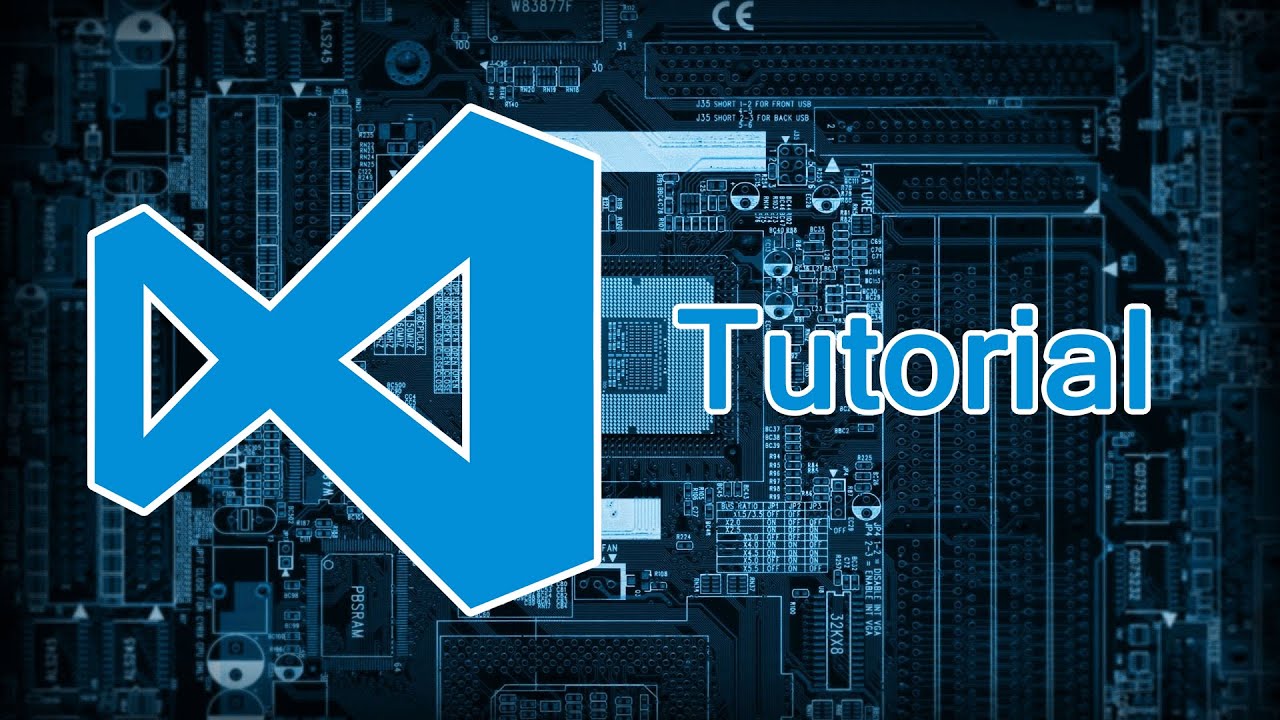
- #Visual studio code python pip how to
- #Visual studio code python pip install
- #Visual studio code python pip windows 10
- #Visual studio code python pip download
#Visual studio code python pip install
To install the Anaconda distribution, go to and look for file Anaconda3-4.1.1-Windows-x86_64.exe, which is a self-extracting executable. I also suggest creating a C:\Keras directory to hold installation files for TensorFlow and Keras. These are all quite stable, but because TensorFlow and Keras are relatively new and under continuous development, by the time you read this article there will likely be newer versions available.īefore starting, I recommend you uninstall any existing Python installations you have on your machine, using the Windows Control Panel, Programs and Features. At the time I'm writing this article, I'm using Ananconda3 4.1.1 (which contains Python 3.5.2 and NumPy 1.11.1 and SciPy 0.17.1), TensorFlow 1.4.0 and Keras 2.1.4. Almost all the installation failures I've seen have been due to version incompatibilities. Installation on Mac and Linux systems is similar.Ĭoordinating compatible versions of Python, auxiliary packages, TensorFlow and Keras is a significant challenge.
#Visual studio code python pip windows 10
In this article I address installation on a Windows 10 machine. I strongly recommend using the Anaconda distribution of Python, which has all the packages you need to run Keras with TensorFlow. Although it's possible to install Python and the packages required to run Keras separately, it's much better to install a Python distribution. First you install Python and several required auxiliary packages such as NumPy and SciPy, then you install TensorFlow, then you install Keras. Installing Keras involves three main steps. All normal error checking has been removed to keep the main ideas as clear as possible.
#Visual studio code python pip download
The source code and the data file used by the demo are also available in the download that accompanies this article. The complete demo code is presented in this article. This article assumes you have intermediate or better programming skill with a C-family language but doesn't assume you know anything about Keras or TensorFlow.
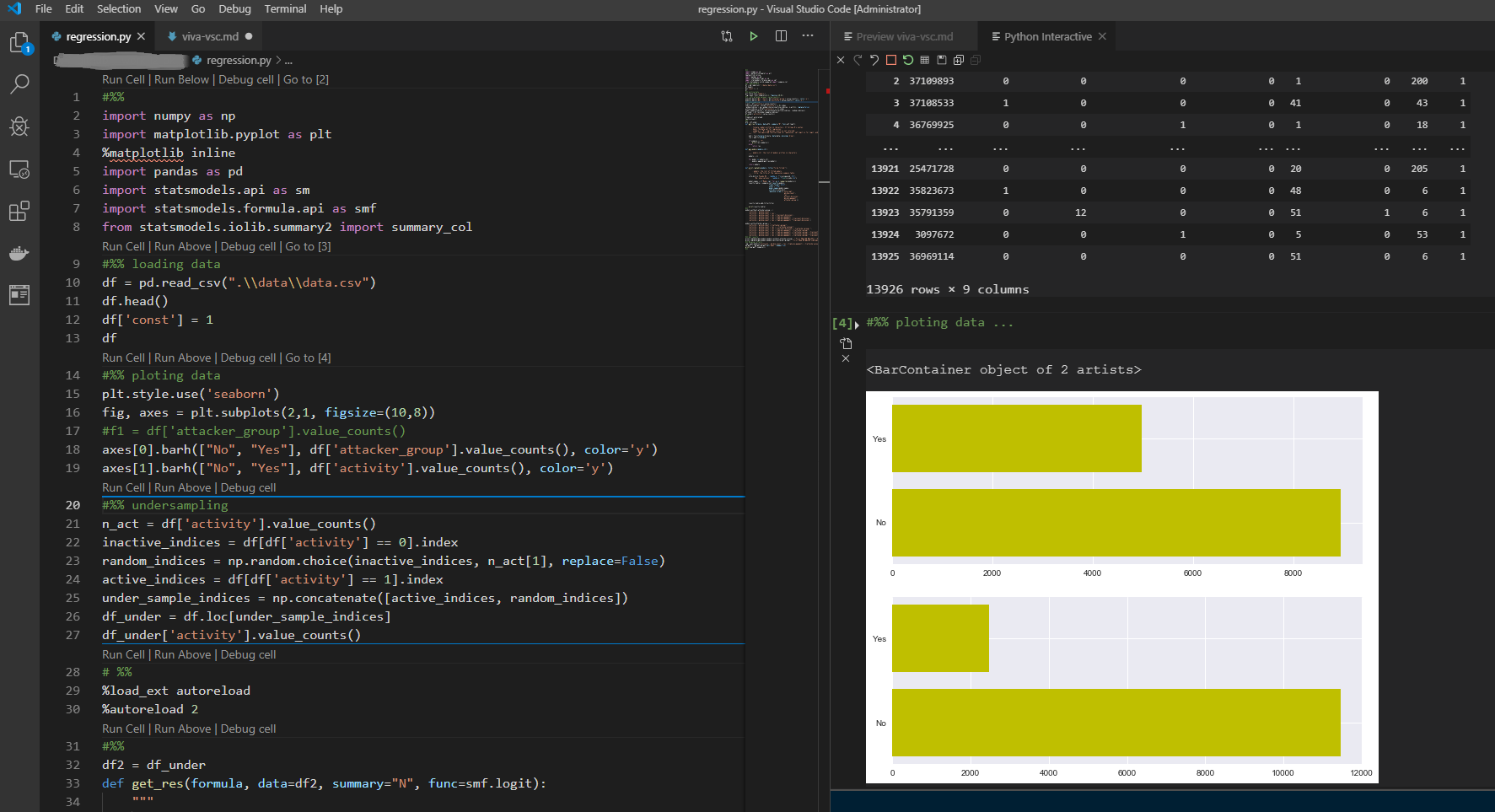
The demo then uses the trained model to predict the species for a flower that has sepal and petal values (6.1, 3.1, 5.1, 1.1). The model has a loss of 0.2703 and the accuracy is 92.67 percent, which means the model correctly predicts the species of 139 of the 150 items. The demo program creates a neural network and then trains the network using 12 epochs (iterations). Figure 1.The Iris Dataset Example Using Keras The goal is to predict the species of an iris flower (setosa, versicolor or virginica) from four predictor values: sepal length, sepal width, petal length and petal width. The demo program reads the famous Iris dataset into memory. However, Keras is used most often with TensorFlow.Ī good way to see where this article is headed is to take a look at the screenshot of a demo program in Figure 1. Interestingly, Keras has a modular design, and you can also use Theano or CNTK as backend engines.

In Keras terminology, TensorFlow is the called backend engine. The Keras code calls into the TensorFlow library, which does all the work. Put another way, you write Keras code using Python. The idea is that TensorFlow works at a relatively low level and coding directly with TensorFlow is very challenging. Keras is a bit unusual because it's a high-level wrapper over TensorFlow.
#Visual studio code python pip how to
In this article I'll show you how to get started with the popular Keras library.

Most neural network libraries are written in C++ for performance but have a Python API for convenience. These libraries include Microsoft CNTK, Google TensorFlow, Theano, PyTorch, scikit-learn and Caffe. But there are many code libraries you can use to speed up the process. It's possible to create neural networks from raw code.
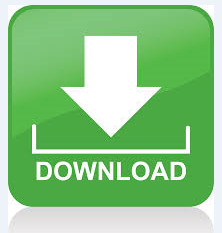